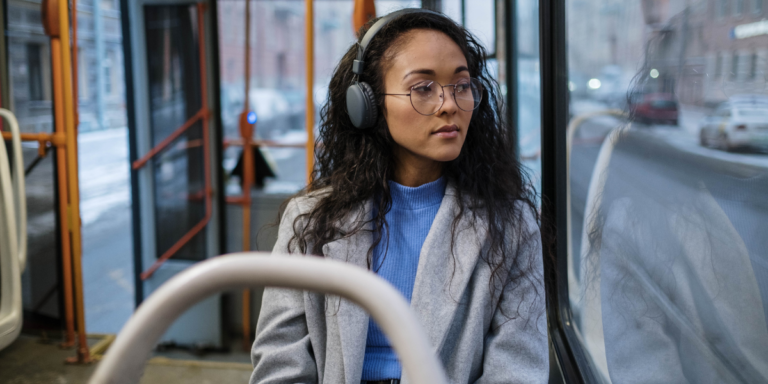
The Case for Running Audio Ads for Your Business
You might hear some people say that audio is dead. We’re here to prove, audio is very much alive and kicking. Seriously. And it’s uniquely
Didn’t think I would do it. Did you? Well, my name is Jeromy and I am in the advertising hot seat today. It’s a hot tub. Could be a sauna in the future. I don’t know. We’ll see. Point is to just get really, really hot and answer questions about advertising. So today we’re gonna talk about something near and dear to my heart, but not actually really.
It’s called ML Overfit and I wouldn’t say it’s near and dear to my heart, but it’s definitely something that comes up a lot in my life.. Both as a media buyer and as a person that now runs an ad platform decibel ads.com. You should check it out. And probably good test budget for audio is at least a million dollars I’d say for month one for every kind of brand.
So just go ahead and start with that and we could scale up after we prove it works. So, ML overfit, what is it? What does it matter to you? All of those sorts of things. ML Overfit is, okay, let’s take a step. When you deploy machine learning you have to train it on a model, right? You have to know what the outcomes are.
You have to know what the underlying training set is, and this kind of gives the machine learning, algorithmic optimization, the ability to then turn around and say, Hey, okay, this is what I am trying to optimize for, right? Like conversions, whatever kind of outcome. Here’s what overfit is. It’s essentially the idea that you can train the model too well, and it will only look for the exact same thing that it was trained on, right? So if you’ve ever run like a meta ad and you have an audience of 10 million people and it zooms in on 10,000 and it just cranks the frequency super high the ads work really well out of the gate and then performance falls off super quick.
You might be experiencing ML overfit, right? Why does ML Overfit matter? And so you see people talk cost caps, bid caps, turn it on, turn it off, teach the algorithm, trick the algorithm, all sorts of absolute just nonsense going around in the Twitter sphere, right? Not that these things don’t work, not that they don’t matter.
They can definitely affect performance outcomes. But it’s too simplistic of an analysis, and if you have too simplistic of an analysis, you’re not gonna be able to actually understand what’s going on. So what’s happening in some cases, not all cases. I don’t work at Meta.
I’ve never worked at Meta. I don’t know what their machine learning looks like. I am a layman. I am not an ML engineer. I am simply a guy that’s been a user and now builds a product with this stuff. Basically you’re potentially running into is the model over optimizing, right? And why that matters is you know, somebody that’s 1% different than the model.
3%, 5% different than the model, right? Could be a really great customer, but the machine learning is actually Ignoring that there’s not enough tolerance, wiggle room for it to actually expand outwards over time. Right? That’s why this matters. And that’s why these little tricks do sometimes work, right? Because it’s overfitting to the model.
And I see a lot of folks, they break down their funnel super granularly and only upload very specific pixel targets that they build lookalikes off of, or upload very specific custom audiences, et cetera, et cetera. That’s fine. But you’re potentially. Looking for ML overfit, right? When you do those sorts of things.
So don’t be afraid to be a little bit more broad. Yes, algorithmic optimization is good. Even with overfit, it tends to outperform humans on average. Not always though. And so when you reset a campaign, when you change up the parameters and you start to get like performance again, that’s what ML overfit is.
That’s why it matters. And as the end user, there’s not a ton you can do about it except for. Throw these little switches and levers. But you can probably do things like give it a more diverse data source, right? You know that it can pull from. Give it more complete information so that it can expand out so that you aren’t overfitting the model.
That way you can find, expand out, find not just 10,000, but a hundred thousand potential customers. You’re not overfitting too hard and you’re not missing out on opportunities driving up your cost per acquisition over time. You know, all of the, that good stuff. That’s why ML Overfit matters to advertisers.
And this has been the advertising hot seat.
You might hear some people say that audio is dead. We’re here to prove, audio is very much alive and kicking. Seriously. And it’s uniquely
Music industry expert Scott Orr has been producing, releasing and promoting albums for more than 10 years, and helping people launch their own indie record
Independent synthwave artist Jay Diggs has 75,000 followers on Instagram thanks to his remixes of pop songs and pop culture. He joins The Business of
No minimum spend or credit details required.
By clicking Subscribe you’ll receive occasional emails form Decibel.
You always have the choice to adjust your interest settings or unsubscribe.